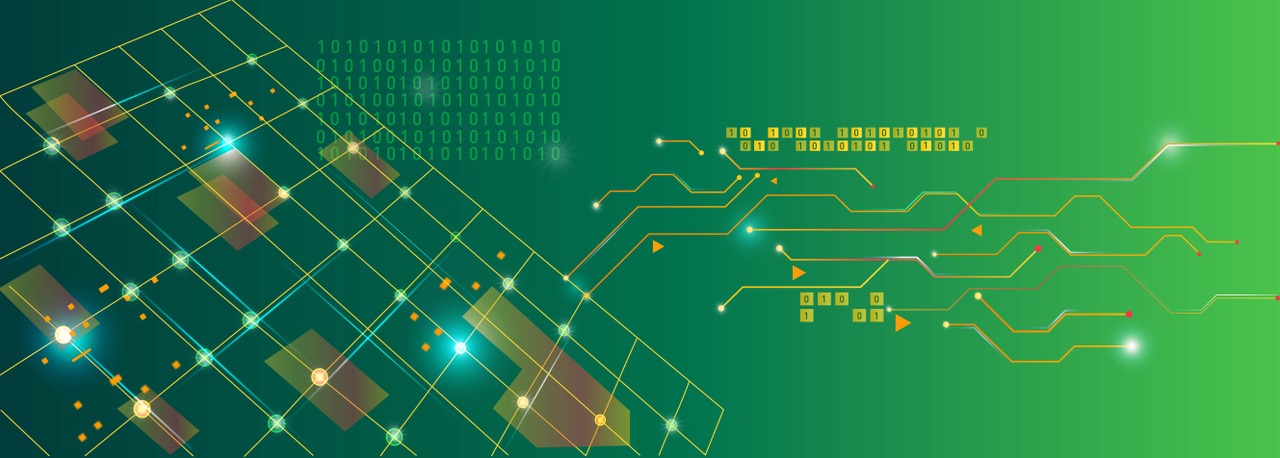
Solar Technology Researcher Becomes an Inaugural Pivot Fellowship Recipient
New Simons Foundation Program Expands Professor’s Work to Machine Learning Techniques
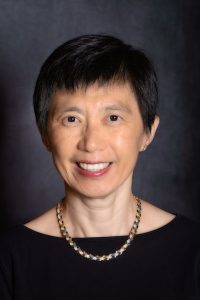
Dr. Julia Hsu, professor of materials science and engineering and holder of the TI Distinguished Chair in Nanoelectronics at The University of Texas at Dallas, studies experimental materials science, particularly new materials for solar cell technology. Through the Simons Foundation Pivot Fellowship, Hsu has expanded her research to include machine learning techniques as she improves the efficiency of solar cell technology.
“It is a tremendous honor for Dr. Hsu to be selected in the inaugural Pivot Fellow Class,
said Dr. Manuel Quevedo-Lopez, head of the Department of Materials Science and Engineering and holder of the TI Distinguished University Chair in Nanoelectronics. “She is one of seven researchers selected from all disciplines across the nation. In addition to the honor it represents for Julia, it is also a tremendous honor for UT Dallas as her selection brings recognition to the University.
“Materials science is highly multidisciplinary and constantly changing. This fellowship has allowed Dr. Hsu to find additional research opportunities outside traditional avenues while providing additional skills that are not normally available to faculty.”
Hsu studies how processes and materials can help substantially increase the efficiency and reduce the cost of solar panel production through her lab located in the Erik Jonsson School of Engineering and Computer Science. However, in the age of machine learning, she wondered if she could better optimize her testing process. She landed a Pivot Fellowship from the Simons Foundation, a new fellowship launched in 2022 that allows accomplished researchers to expand their work to a new field in mathematics or natural sciences for at least a year.
“The Pivot Fellowship has provided an opportunity for me to learn a completely new field,” Hsu said. “In my case, I chose machine learning for experimental optimization.”
Throughout the fellowship, Hsu has worked with Dr. Tonio Buonassisi, a mechanical engineering professor from the Massachusetts Institute of Technology who has used machine learning techniques for solar photovoltaic development.
“Having a mentor who is an expert in the field speeds up the learning curve,” Hsu said. “My mentor pointed me to relevant literature, taught me the language used in the field, pointed out the subtle differences between similar-sounding methods and suggested more efficient ways to accomplish what I want to do. He also generously let me access his postdoc and graduate students who can answer more detailed questions.”
Halide perovskite solar cells are made with a class of materials that have crystalline structures and may be substantially more affordable to produce than current silicon-based photovoltaic solar cells. The United States Solar Energy Technology Office (SETO) has identified three major barriers to the widespread use of perovskite solar cells including stability and durability, module efficiency of or scalability and manufacturing.
“It is a tremendous honor for Dr. Hsu to be selected in the inaugural Pivot Fellow Class… it is also a tremendous honor for UT Dallas. Materials science is highly multidisciplinary and constantly changing. This fellowship has allowed Dr. Hsu to find additional research opportunities outside traditional avenues while providing additional skills that are not normally available to faculty.”
Dr. Manuel Quevedo-Lopez
Head of the Department of Materials Science and
Engineering and TI Distinguished University Chair in Nanoelectronics
Hsu is working on improving the production of flexible perovskite solar cells using a novel photonic curing process that uses short blasts of high-intensity light and eliminates the need for a costly process at high temperatures. In order to find the right process, however, researchers need to explore multiple variables in the laboratory, which can be costly and time-consuming.
While many machine learning methods require a large volume of data to educate a system, Hsu needed a method that works with a smaller data set.
“Machine learning is very broad,” Hsu explained. “We focus specifically on Bayesian optimization with a Gaussian process, which works well for small numbers of data points. Other machine learning techniques, like neural networks or large language models, generally require a large amount of data.”
Hsu’s group recently published some of their findings in Solar Energy Materials and Solar Cells this year.
“My group mostly did the experimental work,” she said. “It provided a gateway for us to learn about machine learning. After learning about Bayesian optimization, my approach to doing experiments has changed. I now immediately think about all the possible variables that can affect the experimental outcome and how to sample them efficiently.”
While machine learning provides important insights into which variable Hsu’s group should test next, Hsu wants to ensure that the results conform to the real world.
“We are applying similar approaches to make transparent conducting electrodes, analyzing spectroscopic data and solving other obstacles we encounter when doing experiments,” Hsu said. “One important thing to remember is that when doing experiments, we are bounded by physical reality and experimental tools. We cannot arbitrarily vary the parameters even when the model tells us to keep going.”
As a bonus, the Simons Foundation also offers the opportunity for Hsu to apply for a $1.5 million grant at the end of her fellowship. With the help of her mentor at MIT, Hsu is now planning where she will go next in her new field of machine learning.